The human brain is superior and more efficient than artificial intelligence, scientists say
From 'The Terminator' to 'I, Robot', killer robots have been a staple of science fiction blockbusters for years.
But scientists say the nightmares of AI overtaking humanity may be further away than we thought.
New research from the University of Oxford suggests that the human brain learns information in a fundamentally different and more efficient way than machines.
This allows humans to learn something after seeing it once, while AI needs to be trained hundreds of times on the same information.
Unlike AI, humans can also learn new information without disrupting the knowledge we already have.
From The Terminator (pictured) to I, Robot: killer robots have been a staple of science fiction blockbusters for years. But scientists say the nightmares of AI overtaking humanity may be further away than we thought
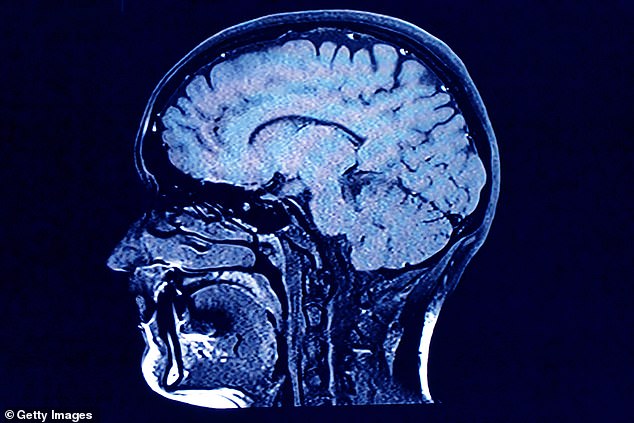
Scientists have discovered that the human brain works in a fundamentally different and more efficient way than AI (stock image)
One of the fundamental learning processes is something called “credit allocation.”
When we make an error, the credit assignment tries to find out where in the information processing pipeline the error was introduced.
Most modern AI consists of artificial neural networks, which are layers of 'nodes' or neurons similar to what you find in the brain.
When an AI makes a mistake, it adjusts the connections between these neurons, known as adjusting the “weights,” to fine-tune its decision-making processes until it gets the right answer.
This process is called backpropagation because errors propagate backward through the AI's neural network.
Until recently, many researchers also thought that this was how biological neural networks such as the human brain learned from new experiences.
In their paper, published in Nature Neuroscience, the authors write: 'Backpropagation, as a simple but effective credit attribution theory, has enabled remarkable advances in the field of artificial intelligence since its inception and has also gained a predominant place in understanding learning in the brain.'
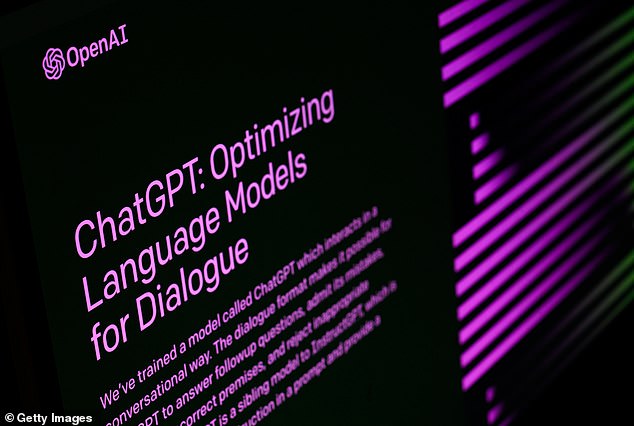
AIs like ChatGPT use a learning method called backpropagation, which adjusts the connections between the 'neurons' every time an error is made
However, they also point out that the brain is superior to AIs that use backpropagation in some important ways.
While AI can outperform humans on tasks from creative thinking to hiring, it takes a long time for AI to learn.
Humans can learn from a single instance of a new experience, while AI needs to be exposed to examples hundreds, if not thousands, of times.
And very importantly: when people learn something new, it does not hinder what we already know, while this is the case for AI.
Faced with this evidence, the researchers looked at the sets of equations that describe how the behavior of neurons in the brain changes.
When they simulated these information processing methods, they found that this was a completely different way of learning backpropagation, which they call prospective configuration.
Unlike AI, which first adjusts the connections between the neurons, the neurons' activity changes so they can better predict the outcome, then the weights are adjusted to fit this new pattern.
While this may not seem like a significant difference, the effects can be very significant.
As an example, the researchers describe a bear that goes fishing.
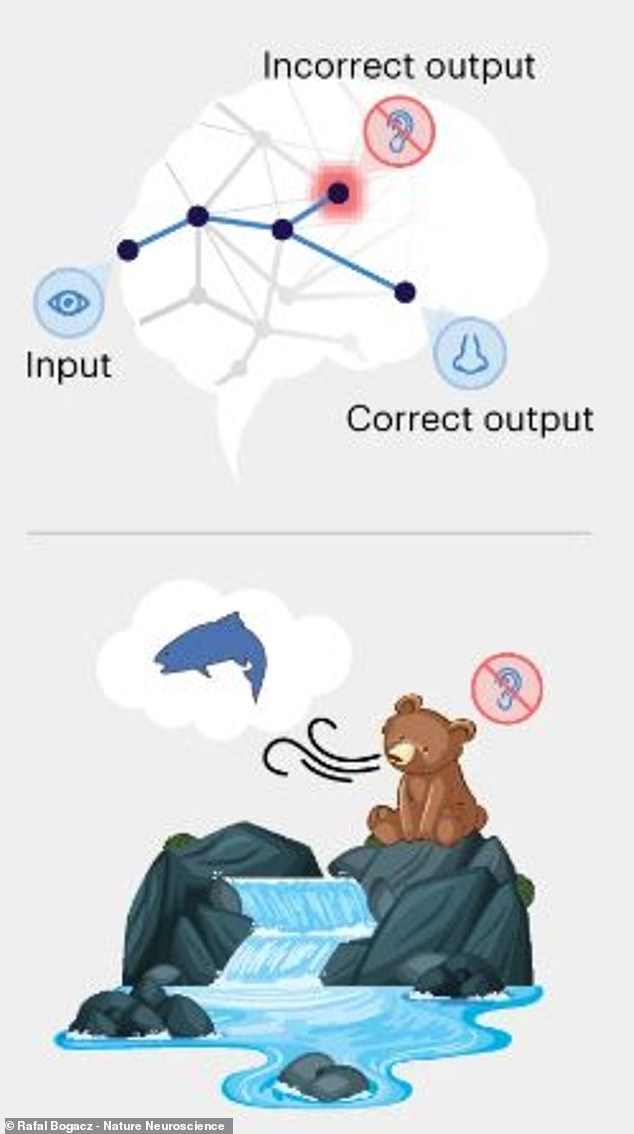
In one example, the researchers explain how a bear will still predict that it should be able to smell fish even if it cannot hear the river as it normally can.
When the bear sees the river, its mind generates predictions about hearing the water and smelling the salmon.
If this works well, the bear may conclude that it should be able to smell salmon in the river when it sees it, and therefore know where to hunt.
But one day the bear comes fishing and hurts his ear, so he can no longer hear the river at all.
If the bear were to use an AI-like learning method, backpropagation would direct this error – the lack of hearing – to reduce the connection between seeing and hearing the river.
But this would also reduce the weight between seeing the river and smelling the fish.
The bear would then be unable to predict that it could smell the fish when it entered the river, and would therefore conclude that there were no salmon in the river.
However, this is clearly not how biological beings work.
Future configuration methods, on the other hand, would ensure that the change in auditory information does not affect the rest of the bear's knowledge.
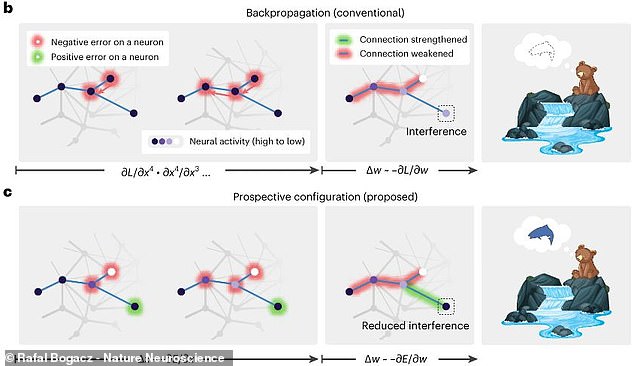
This diagram shows how an AI (top) would predict that there would be no fish if its hearing were lost, while a biological brain (bottom) makes the correct prediction anyway.
But while prospective configuration is a more efficient way of learning, the scientists say current computers can't use these types of systems.
First author of the study, Dr Yuhang Song, says: 'The simulation of future configurations on existing computers is slow because they work in fundamentally different ways than the biological brain.'
Dr. However, Song does say that there is an opportunity to develop new computers that can use this method.
He adds: 'A new type of computer or special brain-inspired hardware must be developed that can implement future configurations quickly and with little energy consumption.'
Lead researcher Professor Rafal Bogacz also points out that there is currently a large knowledge gap between this theory and reality.
Professor Bogacz says: 'There is currently a large gap between abstract models that perform prospective configuration and our detailed knowledge of the anatomy of brain networks.'
He says future research will aim to bridge the gap between algorithms and real brains.